Laura Oliva, (L), is a research associate at University Health Network’s Peter Munk Cardiac Centre and first author of the study. Dr. Lusine Abrahamyan is an assistant professor in the Institute of Health Policy, Management and Evaluation at the University of Toronto. (Photos: UHN StRIDe Team)
Researchers at the Toronto General Hospital Research Institute (TGHRI) used artificial intelligence to analyze administrative health databases and distinguish between patients treated for two different heart conditions.
The study focused on two heart conditions that are caused by an abnormal hole in the upper chambers – the atria – of the heart. These conditions are known as atrial septal defect (ASD), which is a serious congenital heart defect, and patent foramen ovale (PFO), which is found in a quarter of all people and only rarely leads to complications.
“Up to now, distinguishing between patients with these conditions has been difficult due to how they are classified in health administrative databases,” says TGHRI Scientist Dr. Lusine Abrahamyan, who led the research team. “Without population-level data, we are limited in our ability to devise new ways to monitor outcomes and prevent serious complications.”
Both procedures are treated using a minimally invasive procedure known as transcatheter closure. The procedure involves insertion of a catheter through a blood vessel in the groin that is guided up to the heart, where it deploys a small scaffold to plug up the hole.
The research team used machine learning, a type of artificial intelligence, to analyze the health records of more than 4,600 Ontarians who had undergone transcatheter closure between October 2002 and December 2017. The data was provided by the Institute for Clinical Evaluative Sciences (ICES) through CorHealth Ontario, an advisory body to the Minister of Health and Long-Term Care (MOHLTC).
CorHealth is dedicated to improving the quality, efficiency, access and equity of adult cardiac, vascular and stroke services in Ontario, Canada.
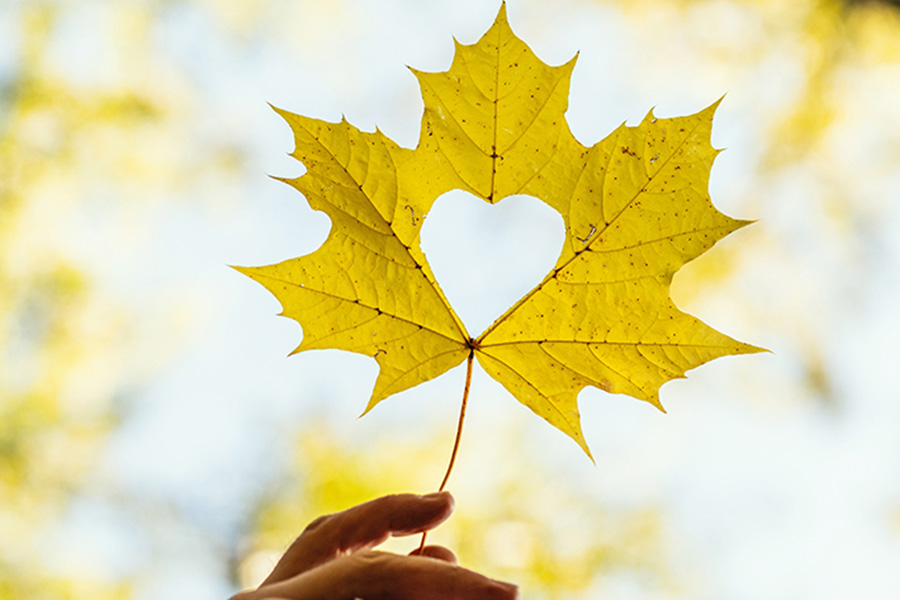
“Our health cards capture each and every encounter we have with health care providers,” explains first author and graduate student Laura Oliva, a research associate working with Dr. Bo Wang, the Lead Artificial Intelligence Scientist at UHN’s Peter Munk Cardiac Centre.
“Each patient was given a unique ICES key number. Using this approach, were able to link this data with national databases of hospital care, community-based ambulatory care and outpatient services in Ontario.”
These databases were then searched for to capture de-identified data for individuals who underwent transcatheter closure.
The research team used a specific type of machine learning approach known as a random forest model to create simple decision trees, which were based on yes or no clinical observations for age, number of ischemic and/or mini strokes and other comorbidities. The most successful model accurately distinguished between the two heart conditions 76 per cent of the time.
“This is the first study to use a machine learning approach to classify patients who have undergone transcatheter closure for PFO or atrial septic defects that has reached this level of accuracy,” explains co-author Dr. Eric Horlick, who is the Peter Munk Chair in Structural Heart Disease Interventions.
“As we refine this approach and link to other datasets, we are getting closer to unlocking the long-term outcomes of these individuals.”
This work was supported in part by donors to UHN Foundation.